Business Analytics Examples: Understanding Practical Applications
Understanding business analytics
Business analytics refer to the skills, technologies, and practice organizations use to investigate past business performance and gain insights that drive business planning. It involves the systematic analysis of data to identify trends, patterns, and relationships that can inform decision make processes.
At its core, business analytics help companies make data drive decisions kinda than rely on intuition or guesswork. This approach has become progressively important as businesses generate and collect vast amounts of data through various channels.
Types of business analytics
Business analytics can be categorized into four main types, each serve different purposes and provide unique insights:
Descriptive analytics
Descriptive analytics answer the question,” what happen? ” iItiinvolvesexamine historical data to understand past performance and behaviors. This type of analytics provide the foundation for more advanced analysis by organize raw data into meaningful information.
Examples of descriptive analytics include:
-
Sales report
That show revenue by product, region, or time period -
Website traffic analysis
That display visitor counts, page views, and bounce rates -
Financial statements
That summarize income, expenses, and profits -
Inventory report
That track stock levels and movement -
Customer demographic data
That profile your customer base
Diagnostic analytics
Diagnostic analytics answer the question,” why did it happen? ” iItddigsdeep into descriptive data to identify the causes of events and behaviors. This type of analytics help businesses understand the factors that influence specific outcomes.
Examples of diagnostic analytics include:
-
Root cause analysis
Of manufacture defects or quality issues -
A / b testing results
That explain why one marketing campaign outperform another -
Customer churn analysis
That identify reasons for customer departures -
Sales performance investigation
That determine why sales increase or decrease -
Supply chain bottleneck identification
That pinpoints cause of delays
Predictive analytics
Predictive analytics answer the question,” what might happen? ” iItuusesstatistical models and forecast techniques to understand the likelihood of future outcomes base on historical data. This type of analytics help businesses anticipate changes and prepare consequently.

Source: intellipaat.com
Examples of predictive analytics include:
-
Sales forecasting
That project future revenue base on historical trends and market factors -
Demand prediction
That estimate future product demand to optimize inventory -
Risk assessment models
That calculate the probability of loan defaults or insurance claims -
Customer lifetime value predictions
That will estimate the total value a customer will bring -
Predictive maintenance
That anticipate equipment failures before they occur
Prescriptive analytics
Prescriptive analytics answer the question,” what should we do? ” iItrrecommendsactions base on predict outcomes. This advanced form of analytics combine predictive models with decision science to suggest optimal courses of action.
Examples of prescriptive analytics include:
-
Price optimization algorithm
That recommend optimal pricing strategies -
Resource allocation models
That determine the best distribution of limited resources -
Treatment recommendation systems
In healthcare that suggest the virtually effective therapies -
Route optimization
For delivery services to minimize time and fuel costs -
Product recommendation engines
That suggest items base on customer preferences
Real world examples of business analytics applications
Retail and e-commerce
Retail businesses leverage analytics extensively to optimize operations and enhance customer experiences:
-
Market basket analysis
Examine purchase patterns to identify which products are oftentimes buy unitedly. Retailers use these insights for store layouts, promotions, and cross-selling strategies. -
Inventory optimization
Use historical sales data and seasonal trends to predict future demand, ensure adequate stock levels while minimize hold costs. -
Customer segmentation
Divide customers into groups base on purchase behavior, demographics, and preferences, allow for targeted marketing campaigns. -
Dynamic pricing
Adjust product prices in real time base on demand, competition, and other market factors to maximize revenue.
Financial services
Financial institutions rely intemperately on analytics to manage risk and improve service delivery:

Source: intellipaat.com
-
Credit scoring models
Assess the creditworthiness of loan applicants base on their financial history and other factors. -
Fraud detection systems
Analyze transaction patterns to identify suspicious activities that may indicate fraudulent behavior. -
Portfolio optimization
Use historical performance data and risk models to suggest investment allocations that balance risk and return. -
Customer attrition prediction
Identify customers at risk of leave, allow proactive retention efforts.
Healthcare
Healthcare organizations apply analytics to improve patient outcomes and operational efficiency:
-
Clinical decision support systems
Analyze patient data to assist physicians in diagnosis and treatment decisions. -
Hospital readmission prediction
Identify patients at high risk of readmission, enable preventive interventions. -
Resource utilization analysis
Optimize staffing levels and bed allocations base on patient flow patterns. -
Population health management
Analyze data across patient populations to identify health trends and risk factors.
Manufacture
Manufacture companies use analytics to streamline production and ensure quality:
-
Predictive maintenance
Monitors equipment performance to predict failures before they occur, reduce downtime. -
Quality control analytics
Identify patterns in defect data to address root causes and improve product quality. -
Supply chain optimization
Analyze supplier performance, transportation costs, and inventory levels to improve efficiency. -
Production planning models
Optimize manufacturing schedules base on demand forecasts, resource constraints, and cost factors.
Marketing
Marketing departments rely on analytics to improve campaign effectiveness and ROI:
-
Campaign performance analysis
Measure the effectiveness of marketing initiatives across various channels. -
Customer journey mapping
Track interactions across touchpoints to understand the path to purchase. -
Attribution modeling
Determine which marketing channels contribute virtually to conversions. -
Sentiment analysis
Examine social media and review data to gauge public perception of brands and products.
Tools and technologies for business analytics
A variety of tools and technologies support business analytics functions:
Data visualization tools
These tools transform complex data into visual formats that are easier to understand and interpret:
-
Tableau
Offer interactive dashboards and visualizations for explore data patterns. -
Power bi
Provide business intelligence capabilities with interactive visualizations and business analytics. -
Looker
Enable businesses to explore, analyze, and share real time business analytics.
Statistical analysis software
These platforms offer advanced statistical capabilities for deeper data analysis:
-
R
Is an open source programming language design for statistical computing and graphics. -
SAS
Provide advanced analytics, multivariate analysis, business intelligence, and predictive analytics. -
Spas
Offer statistical analysis with modules for specific types of analysis.
Big data platforms
These systems handle large volumes of data that traditional databases can not manage efficaciously:
-
Hadoop
Is an open source framework for distribute storage and processing of large datasets. -
Spark
Provide fasting, in memory data processing for large scale data analysis. -
Snowflake
Offer a cloud base data warehouse solution for structured and semi structured data.
Machine learning platforms
These tools enable predictive and prescriptive analytics through advanced algorithms:
-
TensorFlow
Is an open source machine learn framework to build and deploy ml models. -
Python libraries
Like sci kit learn, pandas, andNumPyy provide tools for data manipulation and machine learning. -
H2o.ai
Offer an open source platform for machine learning and predictive analytics.
Implement business analytics in organizations
Successfully implement business analytics require a strategic approach:
Define clear objectives
Organizations should begin by identify specific business problems or opportunities that analytics can address. Clear objectives help focus efforts and resources on initiatives with the highest potential value.
Build the right team
Effective analytics require a mix of technical and business skills. Key roles include:
-
Data analysts
Who collect, process, and analyze data -
Data scientists
Who develop advanced statistical models and algorithm -
Business analysts
Who translate business need into analytics requirements -
Data engineers
Who build and maintain data infrastructure
Ensuring data quality
The accuracy and reliability of analytics depend on the quality of the underlie data. Organizations should establish processes for data governance, include:
- Data cleaning and validation procedures
- Standards for data collection and storage
- Protocols for handle missing or inconsistent data
Create a data driven culture
For analytics to drive value, organizations need to foster a culture where data drive decision-making is value and practice. This involves:
- Train employees to interpret and use data efficaciously
- Make relevant data accessible to decision makers
- Encourage the use of data to challenge assumptions and test hypotheses
Challenges and considerations in business analytics
Despite its benefits, business analytics come with challenges that organizations must address:
Data privacy and ethical concerns
As organizations collect and analyze more data, they must navigate privacy regulations and ethical considerations. This includes:
- Comply with regulations like GDPR, CCPA, and HIPAA
- Implement appropriate data security measures
- Ensure transparent and ethical use of customer data
Integration with existing systems
Analytics solutions oftentimes need to work with legacy systems and diverse data sources. Organizations may face challenges in:
- Connect disparate data systems
- Standardize data formats across platforms
- Manage real time data integration
Skills gap
The demand for analytics talent oftentimes exceed supply. Organizations may struggle with:
- Recruit qualified data professionals
- Develop analytics skills in exist employees
- Retain analytics talent in a competitive market
The future of business analytics
Business analytics continue to evolve, with several trends shape its future direction:
Artificial intelligence and machine learning
Ai and ml are enhanced analytics capabilities by:
- Automate complex analysis tasks
- Identify patterns that humans might miss
- Enable more sophisticated predictive and prescriptive models
Real time analytics
Organizations progressively need insights in real time to respond rapidly to change conditions. This involves:
- Stream processing technologies for continuous data analysis
- Edge computing for processing data close-fitting to its source
- Automated decision systems that can act on insights instantly
Democratization of analytics
Analytics tools are become more accessible to non-technical users through:
- Self-service analytics platforms with intuitive interfaces
- Automated insights that highlight important patterns
- Natural language processing for query data in plain language
Conclusion
Business analytics encompass a wide range of techniques and applications that help organizations transform data into valuable insights. From descriptive analytics that explain what happen to prescriptive analytics that recommend actions, these tools enable more informed decision-making across all business functions.
As data volumes continue to grow and analytics technologies advance, organizations that efficaciously leverage these capabilities gain significant competitive advantages. By understand the various types of business analytics and their applications, businesses can identify opportunities to enhance operations, improve customer experiences, and drive strategic growth.
The examples discuss in this article represent simply a fraction of the ways organizations apply analytics to solve business problems. Whether in retail, finance, healthcare, manufacturing, or marketing, analytics provide powerful tools for turn raw data into actionable insights that drive business success.
This text was generated using a large language model, and select text has been reviewed and moderated for purposes such as readability.
MORE FROM eboxgo.com
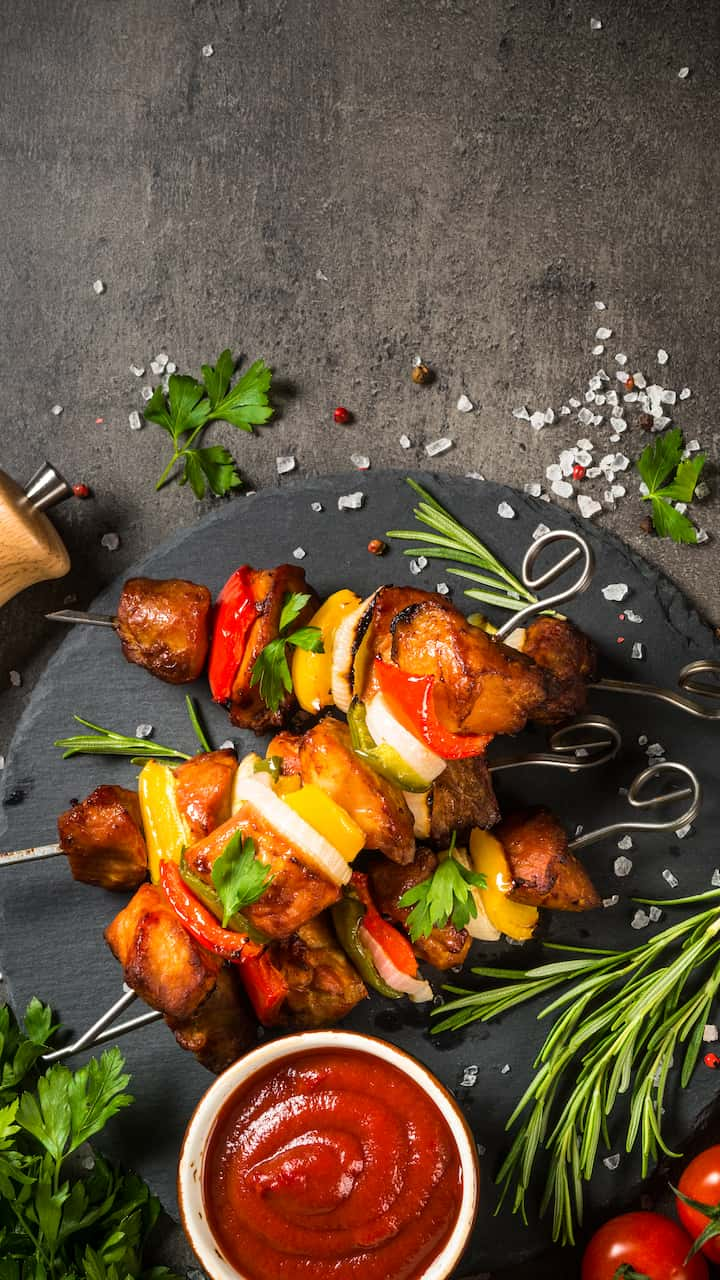
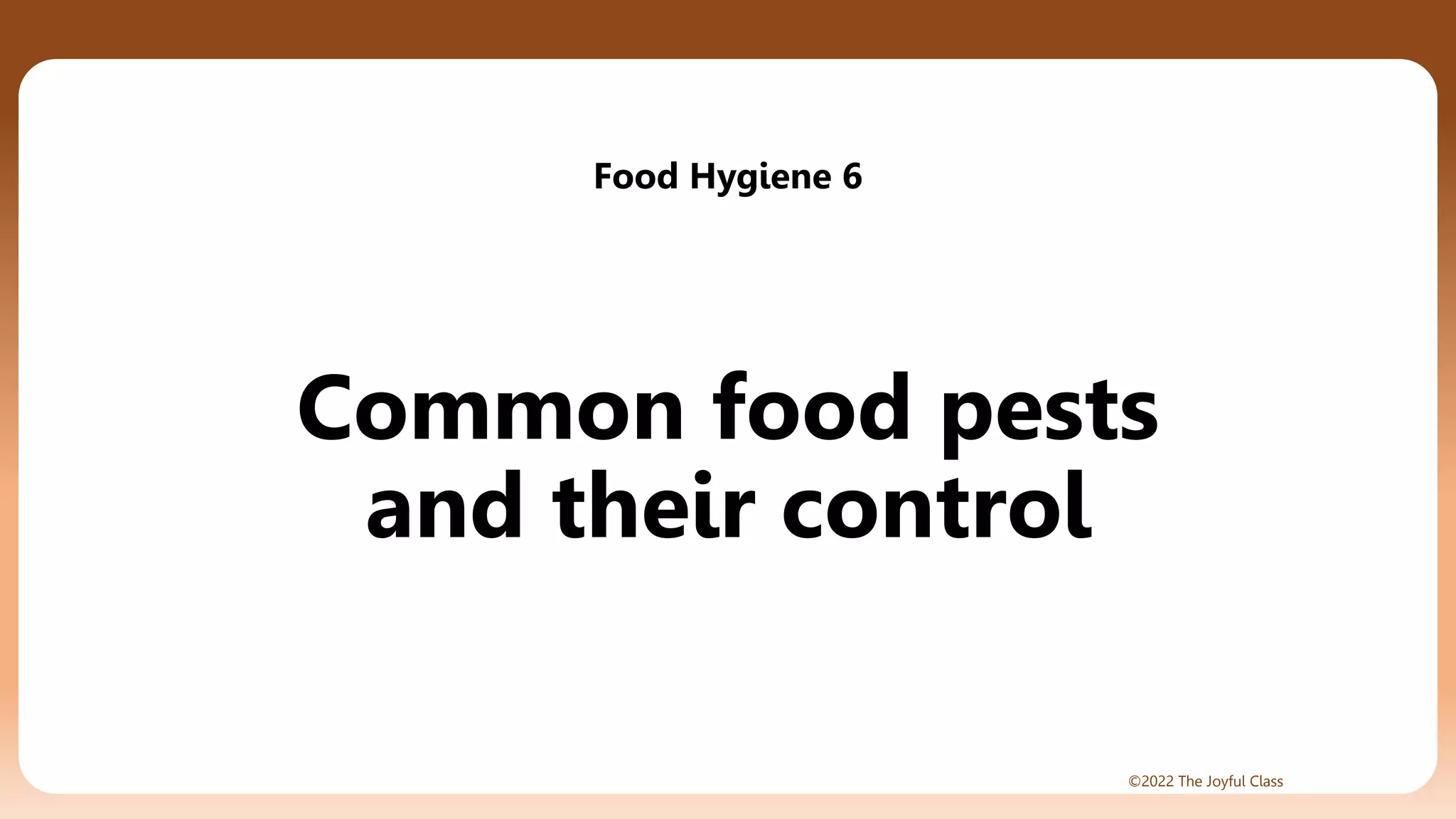
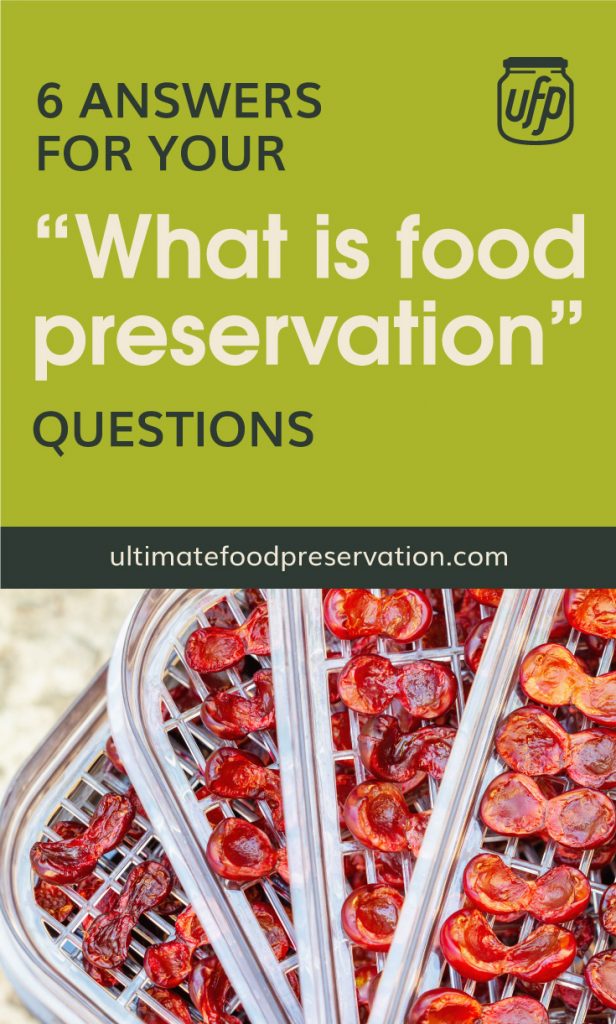
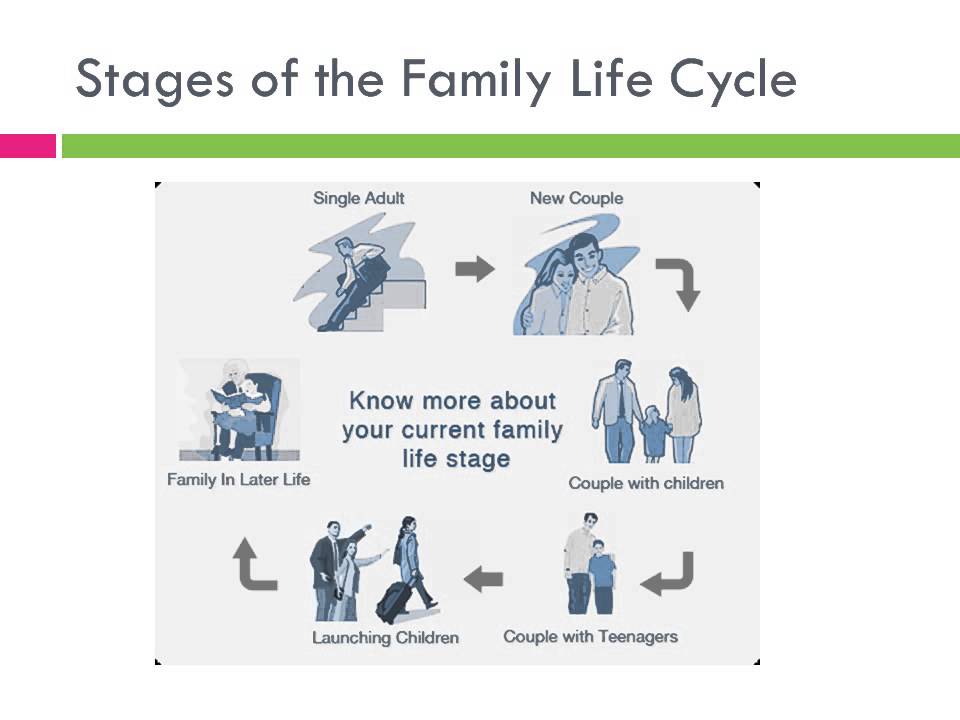
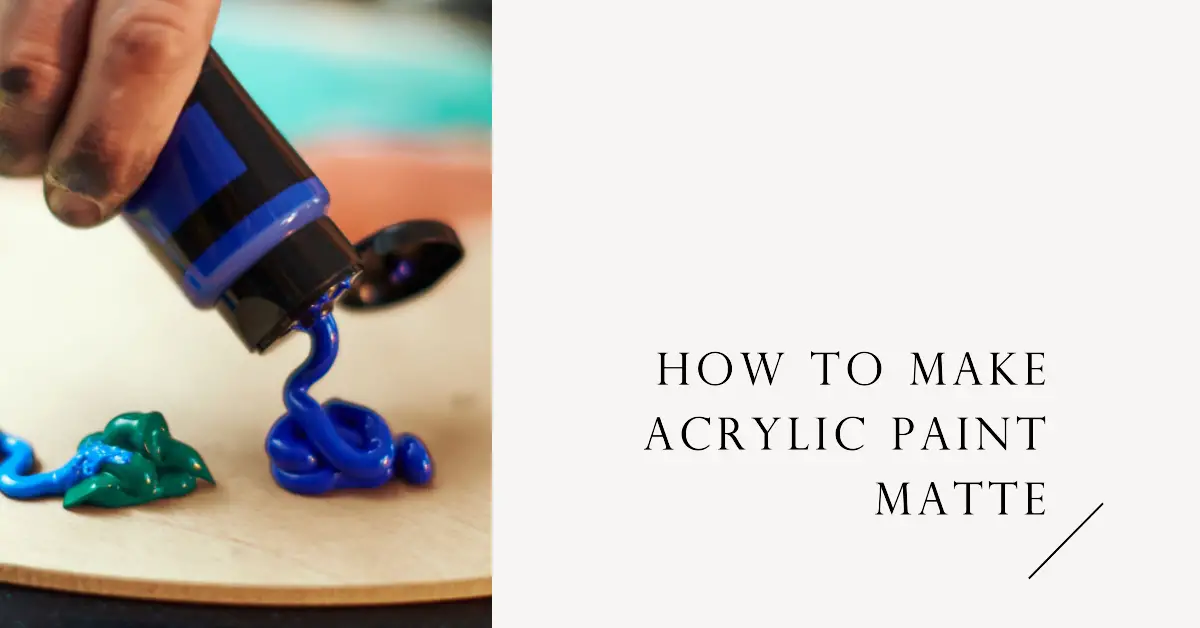
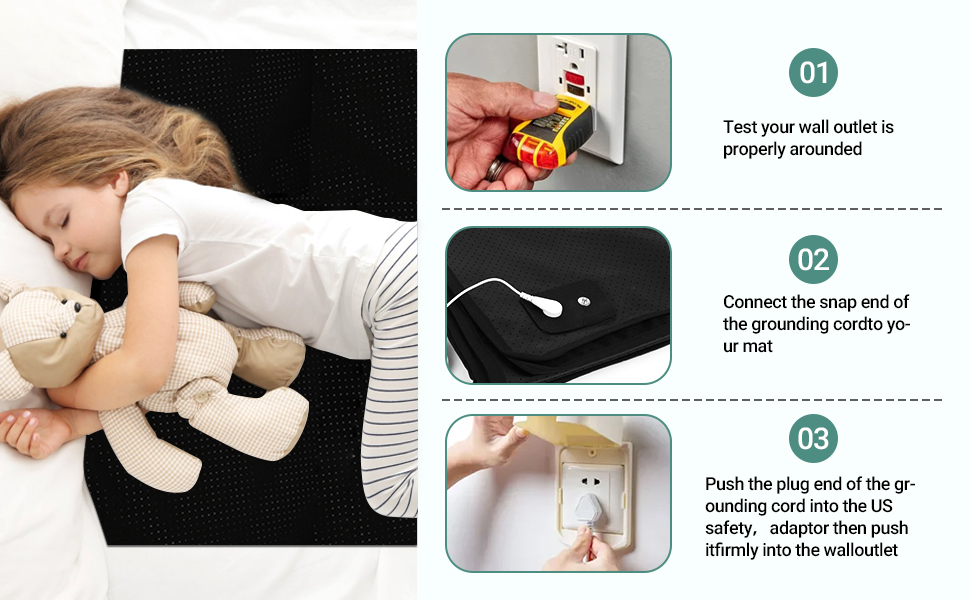
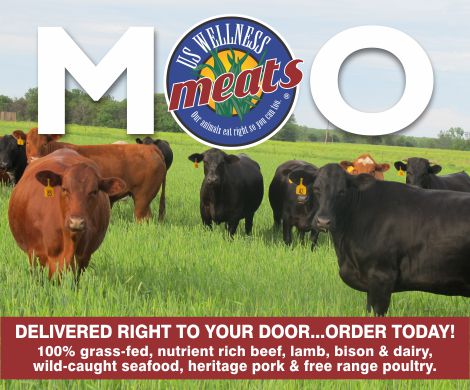
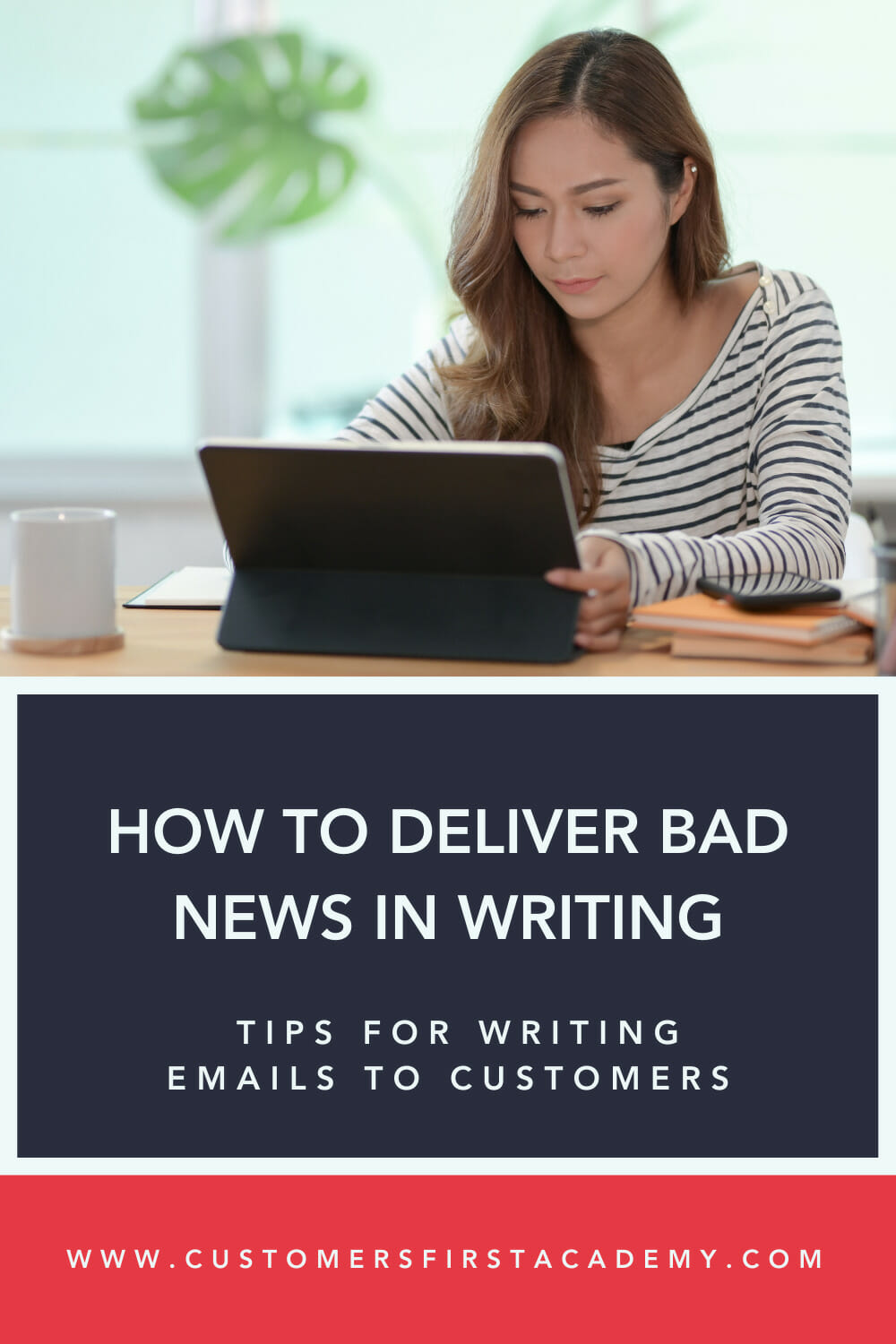
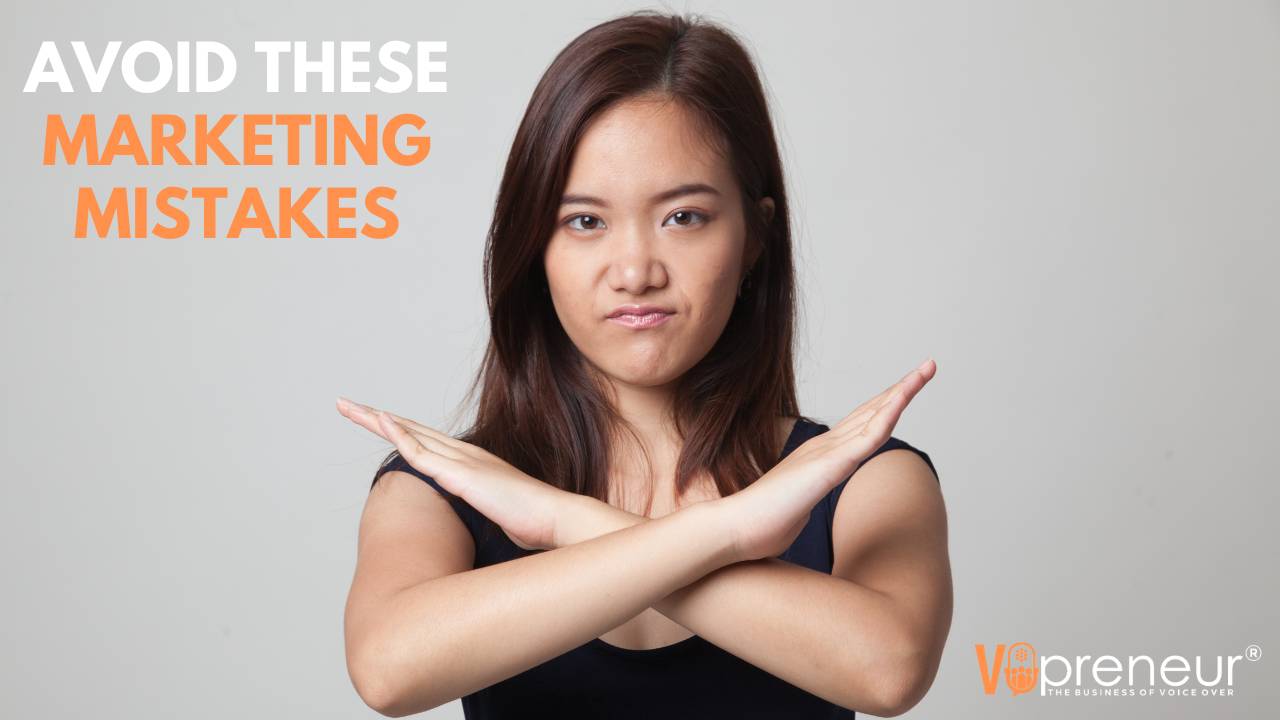
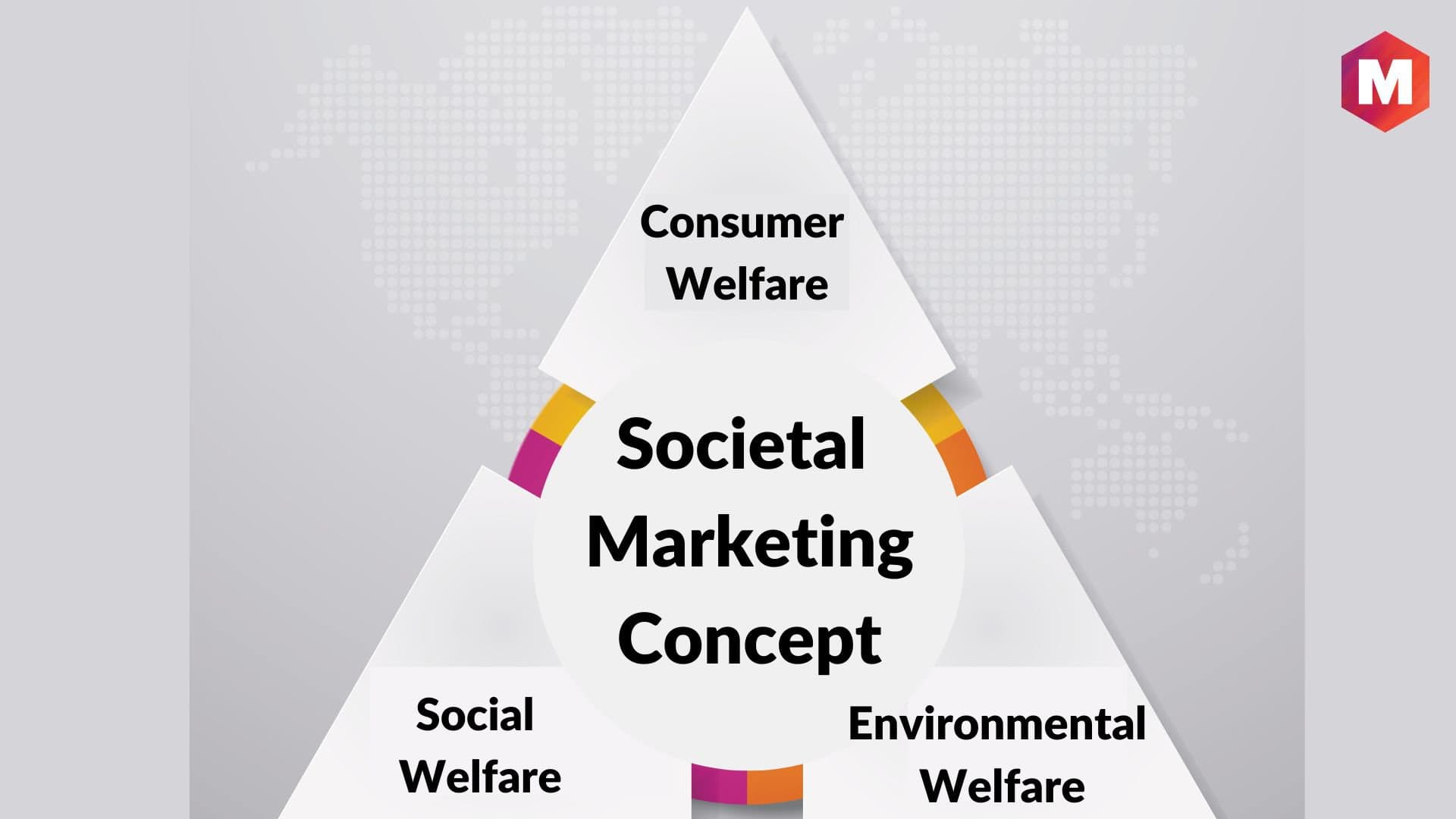
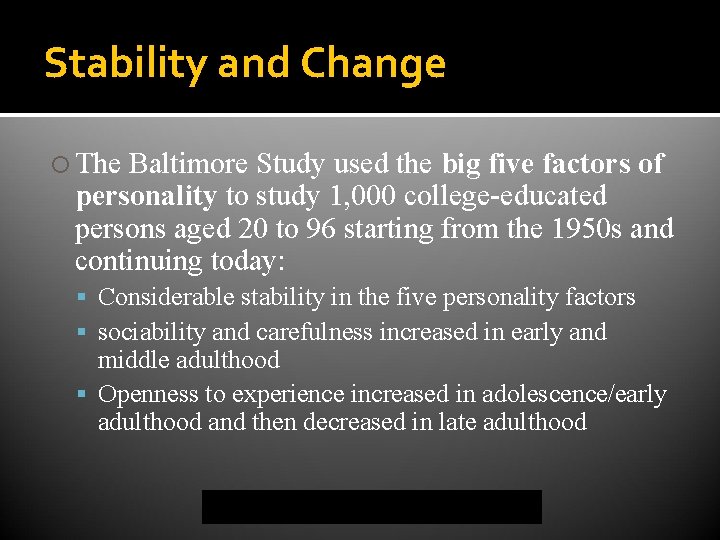
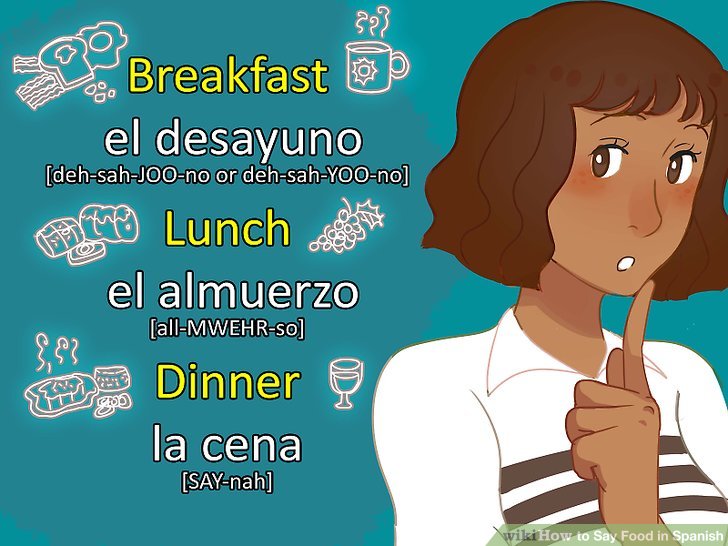